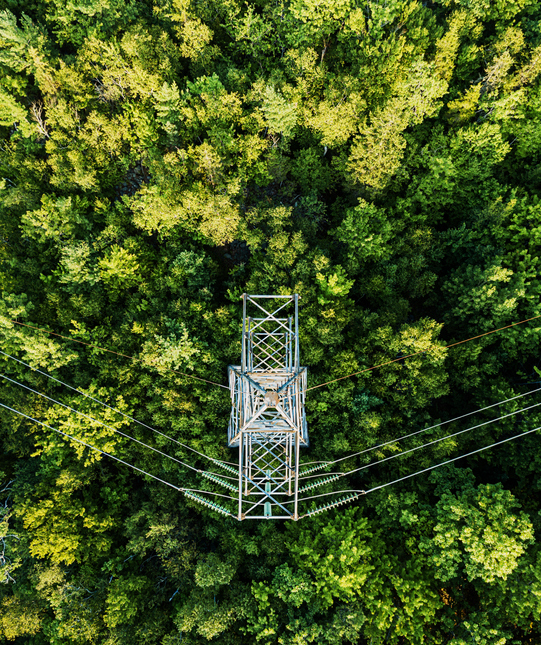
How AI and machine learning algorithms redefine the way utility companies manage their infrastructure
By Jaro Uljanovs, Lead AI Developer and Data Scientist, Sharper Shape
Artificial intelligence (AI) boasts a wide range of potential applications, across nearly every industry imaginable — healthcare, automotive, retail, even fast food. But it’s the utility industry where AI and machine learning (ML) are beginning to demonstrate some of their most impactful effects on many aspects of the business. Power companies are increasingly leaning on AI to improve their electricity delivery and prevent potential wildfires, and AI is actually enhancing, rather than eliminating, human jobs.
From data collection and analysis to their presentation of actionable insights, AI and ML algorithms are quickly redefining how utility companies manage their electric infrastructure.
Consolidating and classifying data
Utility companies oversee massive infrastructure networks, comprising poles, conductors, substations and transmission and distribution lines that span thousands of miles. The vegetation surrounding this key infrastructure must also be monitored, as it presents a danger of fire or outage.
Taking a comprehensive snapshot of these assets means utilizing a variety of different sensors for network inspections. These sensors include lidar, color (RGB), hyperspectral and thermal imagery.
This allows the system to capture everything — from vegetation proximity, to infrastructure assets, to individual components (such as insulators on poles) and their operational integrity, to hot spots indicating potential fire risks.
That’s a lot of data to capture, catalog and process. And there are a lot of individual elements within that data — even in just one image — to pinpoint and classify, let alone do so accurately. Classifying billions of data points across all of those images is an impossibly time-consuming task to do manually.
AI and ML tools can accomplish that same work — scanning thousands of images collected across thousands of miles of utility infrastructure — in seconds. Lidar point cloud segmentation can detect conductors (quite a difficult component-type to segment) with an accuracy of over 90%, while hyperspectral image segmentation can identify vegetation species with an accuracy of up to 99%.
More than that, when paired with drone sensors, these algorithms can also improve the upfront collection of images and data. AI and ML tools help to adjust sensor positioning in real time, in the event a signal is lost or the drone veers slightly away from its inspection flight path.
By helping to readjust the sensors’ bearings while in flight, AI not only ensures more accurate data collection, but also that the flight doesn’t need to be done again or prematurely ended because of faulty data collection, saving time and money. AI pinpoints any faults in the sensors or the drone’s flight path while in the air, recalibrating as needed and identifying individual elements within the data as it comes through the sensor’s video feed.
Breaking down silos to create a holistic data approach
Key to all of this is eliminating the silos that tend to naturally build up between different data segments. In the utility inspection space, asset management, vegetation management, different sensors and so on all produce their own disparate, walled-off sets of data.
When data is kept siloed like this, it becomes unnecessarily difficult if not impossible for teams to derive companywide insights or conclusions from the information being collected. And what good is all that data if it can’t be used to check against itself and enhance other sets of data?
Good data management can’t exist in a piecemeal approach. It needs to be holistic, and AI provides the impetus to make that happen. AI provides a central resource for pooling all these data sources together, making it easier to cross-analyze for potential problems — like wildfire-prone vegetation or damaged components. When these issues are collected in one system, it becomes much easier to identify faults and resolve them — and do so far faster than it would be to manually sift through countless images of poles or vegetation maps.
And for all the stereotypical concerns about AI eliminating work for human beings, at utility companies AI actually enhances the role that people have to play in the network inspection process. Because the AI is what analyzes the data, it’s not something that is dependent on the potentially biased expertise of a professional human inspector, nor is it prone to fatigue and the anomalous results that can come from that. But at the same time, AI can’t do everything itself. It’s a tool for presenting clearer, more accurate and more actionable information for the people to then act on with their own judgment.
There’s a lot of easy-to-make assumptions, both good and bad, about AI. But at the end of the day, what AI really means for the utility industry is a more efficient and effective tool for providing the right information about a power company’s infrastructure — its transmission and distributions lines, its poles, and its nearby vegetation — into the hands of its key decision makers.