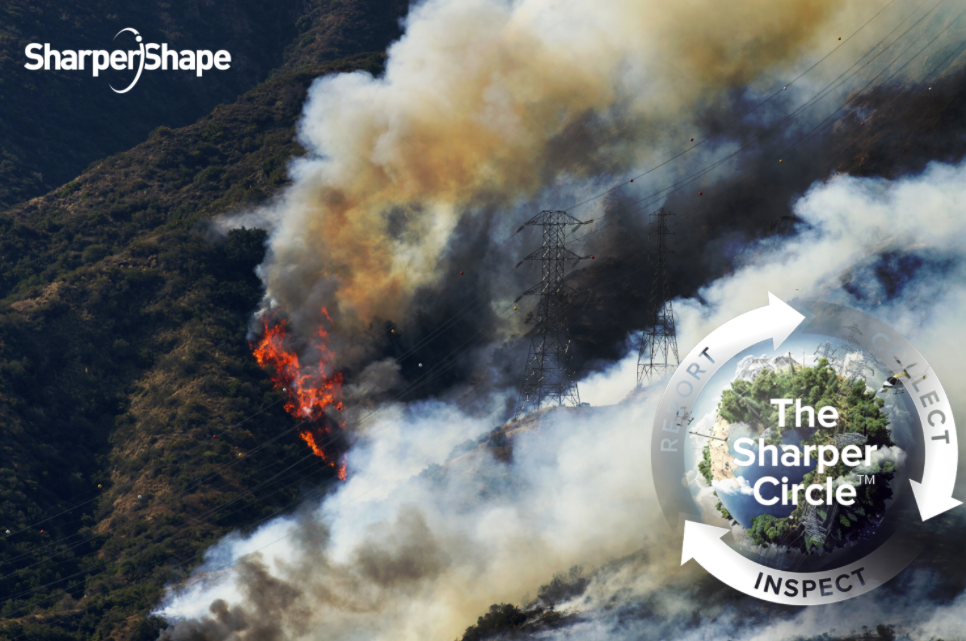
2020 was a terrible year for wildfires. The NIFC reports that 52,934 fires burned 9,539,554 acres in the US, well above the 10-year average of 6,613,017 acres. As climate change will only accelerate matters, electric utilities have a lot to contend with.
During Winter – the wildfire ‘off-season’, though the threat is ever-present – it’s important to assess how utilities can optimize powerline inspection and management in readiness for another fierce wildfire season in summer 2021.
By failing to prepare, you are preparing to fail – Benjamin Franklin
Preventative powerline inspection and management are essential as powerline issues can be a contributor to wildfires.
Traditional inspection methods involve ground crews checking visually for damaged or weakened infrastructure or vegetation management risks. With transmission lines often covering thousands of miles and areas of tricky terrain, it is merely impractical to cover fully on foot. While this is increasingly supplemented with aerial photography and inspections to cover larger regions, the way in which this is often done can incur significant expense.
There’s also the question of thoroughness. These are expertly trained teams, but the human eye has limitations – and as such we should not rely on human examination alone, whether on the ground or in the sky.
Additionally, we need to ensure that the reported information is captured correctly and routed to the right place for remedial action. To maximize preparedness, we need to tackle the effectiveness and thoroughness of data collection; and ways to optimize its value.
Effective preparation requires identification and mitigation of risk factors now, and putting measures into place for rapid response when disaster strikes. An elevated powerline inspection regime provides utilities with a holistic network map and potential risk hotspots, saving vital time in the event of a major blaze.
It always seems impossible until it is done – Nelson Mandela
An optimistic read on the situation is that the severity of the 2020 wildfire season has forced utilities and state and federal governments to take their risk even more seriously. With multiple areas effected, an opportunity arises for greater inter-state and inter-utility cooperation. This enhanced focus should result in increased budgets for wildfire preparedness and response – although, it may take a year to filter into practice.
We can also take a degree of optimism from President Biden’s greater focus on climate change which can only positively influence this context, even if in the longer-term.
But the most significant cause for confidence is technology. Returning to powerline inspection data challenges: the most advanced approaches go beyond the human eye or photography, adding other sensors to the mix, such as LiDAR and hyperspectral imagery, creating a digital twin of the network, enabling data rich and insight-based outcomes.
When combined with advanced software, data analytics are optimized. For example, machine learning techniques combined with LiDAR and hyperspectral data, can map vegetation surrounding powerlines, including identification of fast-growing tree species most likely to cause problems.
Data collection can be time-consuming and costly – particularly when covering larger areas. For example, if a helicopter conducts one flyover to collect HD imagery and another for LiDAR data, the time is at least doubled. However, the most advanced payloads can combine necessary sensor systems to collect all data in a single flight. Another rapidly advancing data collection technique is to use automated drone flights to inspect assets far more thoroughly and quickly than ground-crews.
Though the wildfire threat has never been more significant, modern best practice uniting human and artificial intelligence, supported by the right tools, processes, and partners, enables better options for preparedness than ever before. Sharper Shape’s Sharper Circle of Inspection Automation provides utilities with a holistic process of solutions, combining automated aerial data collection with AI and Machine Learning based inspection analysis and forecasting.